At GCX, we have meticulously engineered our music datasets to address the specific challenges and requirements of AI music generation. Our datasets are not just a collection of audio files and basic metadata; they are a carefully curated and richly annotated resource that enables AI models to understand and generate music with unprecedented depth and sophistication. Let's explore the unique architectural elements that make GCX datasets stand out from the rest.

Multi-Dimensional Metadata:
While most AI music training datasets provide standard metadata like artist, genre, and tempo, GCX takes it to the next level. Our datasets include an extensive range of multi-dimensional metadata that captures the intricate characteristics and context of each music piece. From detailed mood and emotion tags to instrument-specific annotations and performance techniques, our metadata provides a comprehensive understanding of the music, enabling AI models to generate more nuanced and expressive compositions.
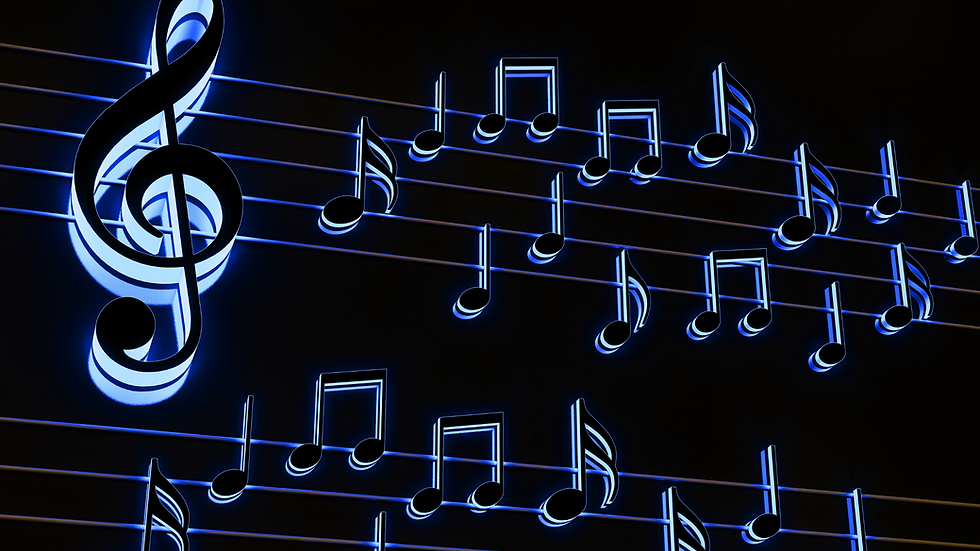
Granular Timing and Chord Information:
One of the key differentiators of GCX datasets is the granularity of our timing and chord information. We provide precise timestamps not just for bars, but for each individual beat within a bar. This granular timing data allows AI models to grasp the intricate rhythmic patterns and micro-timing nuances that are essential for creating humanlike and expressive performances. Additionally, our datasets include detailed chord annotations, specifying the exact chords played on each beat, empowering AI models to understand and replicate complex harmonic progressions with ease.
Expert-Curated Annotations and Voice Prompts:
At GCX, we believe that human expertise is crucial in guiding AI music generation. That's why our datasets feature expert-curated annotations and associated prompts, providing valuable insights and creative direction for AI models. Our team of seasoned music professionals carefully analyzes each track and provides annotations that highlight key musical elements, techniques, and stylistic nuances. These annotations act as a roadmap for AI models, enabling them to generate music that adheres to specific aesthetic goals and creative intentions.
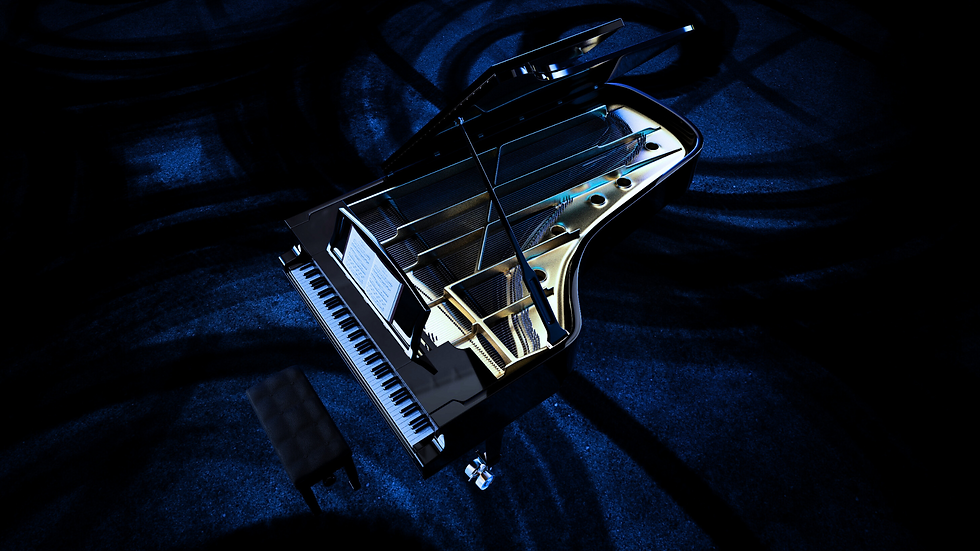
Contextual Data and Cross-Linking:
GCX datasets go beyond isolated musical elements and provide rich contextual data that connects various aspects of music. We establish cross-links between different tracks, genres, and musical eras, as well as mapping the stem, MIDI and sheet music pairs to each track, allowing AI models to understand the relationships and influences between different musical styles and compositions. By leveraging this contextual data, AI models can generate music that is not only technically proficient but also culturally and historically informed.
Iterative Refinement and Expansion:
The architecture of GCX datasets is designed to support continuous refinement and expansion. We actively seek feedback from AI researchers, music professionals, and industry partners to identify areas where our datasets can be enhanced and extended. By incorporating new data points, annotations, and musical insights, we ensure that our datasets remain at the forefront of AI music generation. This iterative approach allows us to adapt to the evolving needs of the AI music community and provide datasets that are always up-to-date and relevant.
By crafting datasets with these unique architectural elements, GCX empowers AI developers and researchers to push the boundaries of AI music generation. Our datasets provide a rich and nuanced understanding of music that goes beyond simple audio files and basic metadata. With GCX datasets, AI models can generate music that is not only technically accurate but also emotionally resonant, stylistically authentic, and culturally informed.
As we continue to refine and expand our dataset architecture, we remain committed to providing the AI music community with the most advanced and comprehensive resources for creating truly remarkable and innovative musical experiences.
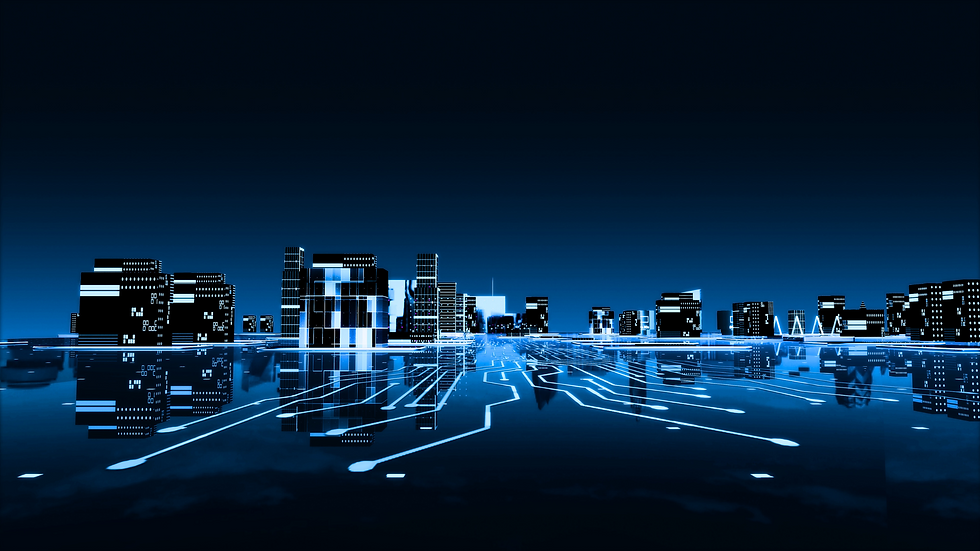